Thursday, October 31, 2024
A Complete Guide to AI Consumer Insights:Ipsos' Best Practices in |001
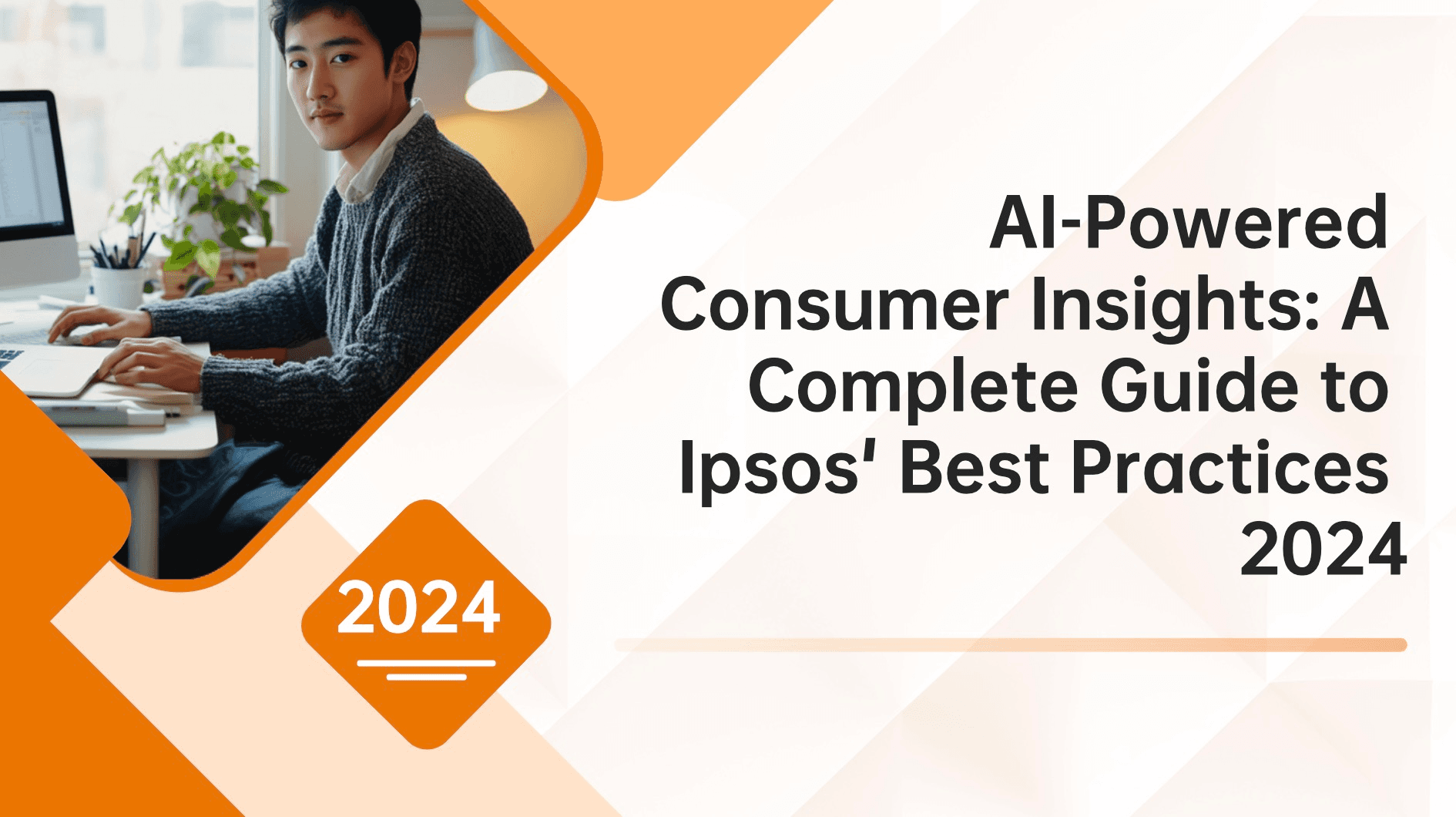
Ipsos is one of the world's largest market research and consulting companies. Mr. Zong Ruixing, as the dean of Ipsos China AI Lab, has over 20 years of experience in data analysis and consumer insights. He is responsible for AI application implementation at Ipsos, continuously driving insight innovation based on artificial intelligence technology for both internal use and clients.
With the rise of generative AI, Ipsos China has been constantly experimenting and breaking through barriers in serving Chinese clients, utilizing AI's capabilities in extraction, summarization, reasoning, and generation to create many innovative and practical marketing insights that help drive business growth. Ipsos is now productizing and developing solutions based on these experiences to serve more insights, product, and marketing professionals.
Zong Ruixing's thoughts on AI insight technology and how to use AI provide great inspiration for introducing AI into marketing:
- AI will transform the insights industry, enabling insights to create greater value in business
- The entire insights process will be impacted by AI, including data collection, analysis, and delivery
- Success in AI insights requires excellence in three areas: data, prompts, and workflows
- AI enables us to analyze more modalities and deeper data
- Simply throwing questions at AI won't yield insights; a good workflow is needed to maximize AI's value
- With AI, businesses receive reports plus consumer digital twins; PersonaBot embeds AI insights into daily operations across departments...
01 Hiring an AI Chat Assistant as a User Research Interviewer
Hu: In this wave of generative AI applications and practices, which scenario or stage of marketing insights has seen the greatest empowerment from generative AI?
Zong: We divide insights into 3 stages: collection, analysis, and delivery. Currently, all three stages are being empowered, but analysis has seen the greatest impact.
Hu: What kind of empowerment can you introduce to us separately?
Zong: First, in the collection aspect, we've made some processes more intelligent and interactive. For example, in our commonly used market research survey systems, we've integrated AI. When we ask consumers questions about whether they like a product or not, we analyze their reasons for liking or disliking in real-time and make automatic follow-up questions.
Hu: Like chatting with an AI assistant?
Zong: Yes, like an AI chat assistant. Market research includes both qualitative and quantitative research. Qualitative research is like us sitting and chatting for over an hour, which can be quite in-depth; quantitative research mainly involves filling out questionnaires, maybe just typing a few words, all very standardized. But with AI functionality, it's like having an interviewer beside you who analyzes your answers in real-time and makes one or two follow-up questions, allowing you to provide deeper results, giving us the opportunity to understand better.
Hu: This is like incorporating qualitative research methods into quantitative research. In the past, such follow-up questions could only be achieved through face-to-face interviews, right?
Zong: In the past, this was typically called offline research, where interviewers used paper questionnaires or tablets for face-to-face follow-up questions, but now with large amounts of online research and online surveys, follow-up questions weren't possible. Now with AI, we can make follow-up questions like an online interviewer. Consumers' open-ended responses are very valuable, and compared to traditional online surveys, with AI follow-up questions, the results are much richer.
Hu: This is like hiring a Siri or an AI chat assistant to do research. But while chat assistants might be good at asking common, everyday questions, how do you ensure they can ask professional questions deeply and accurately enough?
Zong: First, most of our consumer research is still focused on the consumer goods sector - it's relatively lifestyle-oriented, and current AI's understanding capability isn't a problem. Another important point is that the follow-up questions aren't about answering consumer questions, but rather making deeper inquiries based on consumer responses. For example, if a consumer says they like the appearance, AI will ask about specific aspects of the appearance. This can actually be well addressed through prompts. Even in professional fields, there are no technical difficulties. We prepare these prompts in advance, telling AI how to get more specific feedback for relatively vague answers. So far, it's been quite accurate.
02 AI Makes Consumer Insights Analysis Faster
Hu: In terms of data analysis, what changes has AI brought?
Zong: Data processing and analysis are the most time and labor-consuming stages for research teams. Among the various consumer insights processes, AI has currently made the biggest impact in the analysis segment.
First, AI makes analysis faster. When we conduct analysis, we need to process textual data and categorize it through coding. We have a system where the typical workflow is as follows - analysts first input a batch of text data for dialogue and define the labels; after defining them, they then put all the text data into our system to tag each entry.
Hu: In the past, analysts indeed spent a lot of time on this part, repeatedly defining labels, correcting, adjusting...
Zong: Yes. AI excels particularly at categorization. It can define labels quickly and relatively accurately, including label naming and explanations. With AI now, all of this can be done very quickly, and analysts only need to make certain corrections. Then we input these names and explanations into the system, throw in the text data, and results come out quickly. From what we've seen so far, the results are very good. So, AI can make our analysis much faster.
03 AI Can Analyze More Modalities of Consumer Data
Hu: Besides being faster, what else?
Zong: Second, AI can analyze more data. Before AI, some text data was very difficult for us to analyze. With AI, we can now do deeper analysis across more modalities. Last year we did a case study right when AIGC*()* first came out - we helped a restaurant client innovate their menu items. Our approach was to innovate through studying food culture, since food culture isn't just about good taste - it has deeper meaning that reflects regional and national cultural heritage.
Hu: How do you use AI to conduct this kind of analysis?
Zong: Our approach is to analyze narration from food documentaries, like "A Bite of China" and "Taste of Shunde", which comprehensively reflect Chinese food culture. The content is quite diverse - it could be life stories, local legends, or ingredient origins. We use generative AI to extract ingredients mentioned in them. First, we convert video narration into text, then have AI extract characteristics like ingredients, flavors, regions, and stories. After extraction, AI helps summarize step by step. Previously, we rarely analyzed such content because the manual cost was too high. So AI allows us to expand our analysis scope.
Hu: Is this still based on AI's categorization ability?
Zong: First is extraction (information extraction capability), second is categorization ability. We now also use AI to analyze academic articles and papers. For example, when helping clients with R&D to understand technical development trends in a field, previously our team had great difficulty reading highly specialized academic content - lots of technical terms, many in English, hard to understand without professional background. But with AI, we can extract conclusions from academic papers, build a database, then write white papers for clients. Their R&D teams just need to read the white papers. Corporate R&D doesn't need to go that deep - they just need to see current technology development trends.
04 How AI Enables Deeper Analysis of Consumer Insights
Hu: So the second change is that AI can analyze more diverse data, broadening the scope of analysis. Anything else?
Zong: The third change is that AI enables deeper analysis. We've all experienced trying to extract valuable insights from vast amounts of information and feeling overwhelmed - the human brain can only handle so much information. In the past, analysis heavily depended on experienced analysts who would form conclusions in their minds after reviewing raw materials, which would then guide the analysis direction. This was not only time-consuming but often limited in depth. Now, we can use AIGC to help with analysis.
Hu: How do you conduct analysis with AI now?
Zong: Let me give you an example. Recently, we were analyzing and forecasting trends in China's beverage market by studying Japan's market, since Japan is more advanced in this sector. We analyzed award-winning products from a major Japanese food and beverage competition. Each beverage had detailed descriptions, and we used the information extraction method mentioned earlier to identify the characteristics of each beverage. However, there were many beverages with numerous features - functions, benefits, flavors - all without clear patterns, making them difficult to use directly. This is where we needed to synthesize the information.
We had AI distill the main dimensions from these attributes to summarize Japan's beverage innovation directions. AI's summary of innovation directions was excellent. We plotted these summaries on two axes to create a functional matrix of Japanese beverages, then positioned different beverages in various locations, creating a functional map of the Japanese beverage market.
Hu: Compared to before, where do you see the improvements?
Zong: Humans can do this, but it requires highly experienced analysts and takes a very long time. The final dimensions are still determined by humans, not AI, but AI provides numerous insights that experienced professionals can then evaluate. So AI integration allows us to analyze more deeply. For similar analyses - extracting underlying reasons from large amounts of information and abstracting dimensions - we now use AI.
Another interesting aspect is having AI name these dimensions. I find AI's naming to be better than humans - it creates creative, apt, catchy, and engaging names. AI shows impressive creativity in this regard.
05 We Now Deliver Reports Plus Consumer Digital Twins
Hu: So to summarize, AI makes insight analysis faster, broader, and deeper. How does AI enhance the delivery phase?
Zong: In data collection and analysis phases, strictly speaking, AI only enhances and optimizes existing workflows. But in the delivery phase, AI integration becomes interesting because we create new scenarios and new delivery products.
Hu: How innovative is it?
Zong: We mainly serve large clients. Previously, we delivered beautiful PowerPoint reports, but there were two problems: First, reports often went unread; Second, when R&D or Marketing teams used these reports, many details were missing because the final PPT was a highly abstracted result of multiple summaries. So we're now experimenting with what we internally call PersonaBot, or consumer digital twins.
Hu: Consumer digital twins - what does that mean?
Zong: The digital twin concept comes from industry, like Siemens selling engines with accompanying computer models for simulation. We use AI's reasoning and text comprehension abilities to create consumer digital twins.
During our projects and research, we have extensive consumer interactions through interviews, home visits, and shopping accompaniment, collecting vast information about household composition, income, work situation, values, consumption views, and product attitudes. Previously, this information often went unused. Now we use AI to build models that mirror consumers.
Hu: How does this relate to delivery?
Zong: When we conduct projects with dozens or hundreds of user interviews, traditionally we'd write an abstract report based on this research. Now, along with the report, we deliver these digital twin virtual consumers to clients - for example, if we interviewed 10 people, we deliver 10 virtual consumers. These digital twin virtual consumers are modeled based on interview content.
Hu: What are the use cases for these digital twin virtual consumers?
Zong: When you have questions while reading the report and want to understand details more deeply, you can simply ask these virtual consumers and chat with them.
Hu: Another chat assistant?
Zong: It's like deconstructing a PPT - there's a chat box where you can ask anything: why do you like classical style, what do you think about this product... It responds based on previous interview results. We ensure it only answers based on interview content, and AI will respond with "don't know" or "not sure" when it can't answer. We used to provide interview transcripts to clients, but these could be 20-30,000 words per person, making it difficult for clients to read and find key information.
Hu: That's a really interesting use case.
Zong: We can make it even more sophisticated by adding a workflow. If among these 10 consumers there are both young and older people, and you want to know young people's perspectives, a virtual moderator or analyst will ask each digital twin consumer this question, then summarize their responses, giving you a synthesis rather than individual responses.
Hu: So you can get answers beyond what's in the PPT report? That's really value-adding!
Zong: Yes, all answers are based on our sample database and interview results, with extensive expansion. Through AI, we've activated our consumer knowledge base. Our previous PPT reports often contained concepts extracted by researchers to make persuasive points. But when implementing, these conclusions alone sometimes aren't enough, which is where PersonaBot comes in to meet clients' deeper needs.
Hu: Is PersonaBot only applicable for report interpretation?
Zong: We're not just using it for consumer understanding. PersonaBot has other functions, like feedback. What's feedback? You can present an idea and ask consumers how they view it from their perspective. We know large models have strong reasoning capabilities based on facts.
Hu: What are suitable use cases?
Zong: For example, R&D teams can have instant access to consumer feedback. They'll still need to communicate with real people after developing actual products, but with PersonaBot, they can get feedback anytime during daily work.
There are currently some limitations - it can't cover everything since it's not a real person. So we make it quite sophisticated with a workflow system. Simply asking questions directly to a knowledge base with lots of transcripts doesn't work well. We have an AI-driven workflow to control the entire process, fully leveraging AI's technical characteristics to produce high-quality content that reflects consumer needs and provides work insights.
06 How to Integrates AI into Daily Operations Across Enterprise Departments
Hu: Are clients using it? What's their feedback?
Zong: Many companies collect consumer information for product innovation, with new products launching annually. Clients hold yearly workshops and internal brainstorming sessions where team members from different departments review consumer interview records or conduct interviews to analyze user needs and develop new ideas. We've developed PersonaBot to become a virtual team member in these sessions, integrating AI into the entire workshop process to spark creativity. Clients are already using it with excellent results.
Hu: Would it be fair to say that future AI insights will be delivered more as online products or data+products rather than reports?
Zong: I believe there will still be demand for reports. Reports are valuable because they extract highly refined, high-level conclusions from vast amounts of information. Their importance lies in persuasion - showing how we segment populations, what types of users we're dealing with... something the whole company needs to see. This is about management and persuasion. AI tools, being too flexible, can't replace this - they won't significantly impact report delivery formats. AI will provide additional value by helping implement insights alongside reports.
Previously, reports were mainly read by research teams and management, with business teams occasionally attending report interpretations. Now with AI-assisted implementation, it can extend to more teams, like packaging designers and R&D teams, who can ask questions through AI. It can also serve as a real consumer assistant for marketers, helping them understand consumers better.
This represents a crucial new value output that didn't exist before. All departments can now actively use reports, effectively embedding them through AI into their daily work, such as on enterprise WeChat or Feishu, where they can instantly @consumer Zhang San for opinions, or ask AI about young mothers' or students' perspectives on various issues... This is a significant addition.
07 The Biggest Challenge of AI: Accuracy
Hu: What challenges have you encountered in developing AI online insight products like PersonaBot, and how did you solve them?
Zong: The biggest challenge was perception. Generative AI developed rapidly, especially last year when everyone thought AI was omnipotent. But when implementing it, many issues arose that didn't meet expectations. People wondered, "Shouldn't AI be more powerful?" We later discovered we couldn't rely solely on large models. Things like prompts, and especially this year's focus on workflows and agents, are crucial. These additions led to significant improvements. So the main challenge was overall perception of AI applications - once we solved this cognitive issue, we crossed a major hurdle.
Hu: How do you think AI insight products should be developed and structured?
Zong: The AI product architecture has large models at its foundation, where we select the most suitable ones. Above that is the knowledge base, containing not just consumer information but also industry and competitor data. Above the knowledge base is what we call workflow. On top is the chat interface and insight interaction - we're exploring future evolution, possibly including human-like avatars. The key is understanding how the entire AI tech stack integrates with business logic.
For companies like Ipsos, our core advantage is business understanding. Much of this understanding and information existed before, but how it combines with AI to solve business problems is our expertise. We can't just focus on large models - the workflows and prompts are quite complex.
Hu: So you're creating an analyst AI Agent?
Zong: You could say that. We're essentially building an AI Agent, including what we call an LLM Operation System that manages the prompts and workflows we discussed.
Hu: Is workflow the most critical aspect of these products?
Zong: Good AI insights require three conditions: better and fresher data, better prompts, and more scientific and rational workflows. When these three elements are in place, AI insight quality becomes excellent. Simply uploading data and asking AI for new ideas usually produces mediocre results. We need very scientific and detailed workflows. Next year marks Ipsos's 50th anniversary, and in many areas, like product innovation, we have very scientific methodologies that we incorporate into AI processes. We don't just ask for new ideas directly - we first explore possibilities like scenario needs, ingredient analysis, packaging analysis, etc., before combining everything. The process exists within our past experience.
Often we need to define these processes and see how AI can empower them, then let analysts use and provide feedback. These processes can be complex - some workflows might use several AI tools, including visual and language tools, with multiple language models having different characteristics.
Hu: Do you see any barriers regarding foundational large models?
Zong: Overall, we're more application-focused and interface with many large models. Large models are developing and iterating very quickly. Our job is to stay familiar with technical developments and fully utilize their potential.
Hu: When using AI, we often encounter "creative freedom," but insights work requires precision. How do you control AI response quality?
Zong: In our workflow, AI performs two types of tasks. One is creative, where freedom is acceptable as we need new ideas. The other is factual, where we have extensive material-based content. We currently use several control methods: first through knowledge bases, which provide significant control; second through prompts that explicitly tell AI to indicate when information isn't in the knowledge base or to admit not knowing; third by sourcing all information - for example, when analyzing transcripts, each statement shows who said it with clickable source references.
This approach eliminates large model "hallucinations" in factual analysis and prevents fabrication.
08 AI Solutions: A Revolutionary New Logic in Insights
Hu: Besides PersonaBot, what other interesting products or experiments have you developed?
Zong: We also have AI Solutions. AI Solutions combine data, business logic, and scenarios - it's more vertical. For example, our first AI Solution was a product innovation tool for the beverage industry, starting from basic consumer scenarios. The unique aspect of beverage innovation is that it's not based on satisfied needs - current beverage innovation can't just meet stated user needs, it needs to surprise users with unexpected flavors, functions, packaging, or scenarios. These product innovations come from real scenarios, aiming to innovate and surprise users, so we still need to understand consumer drinking scenarios.
Ipsos has consumer communities where we find many consumers to photograph their beverage consumption scenarios and describe them - for example, what they ate and drank during office lunch, their feelings and mood. This creates a database. We then use AI to analyze these scenarios, adding many tags like gathering moments, couple time, or solo moments. This allows us to quickly see different beverage consumption scenarios from hundreds of users across China without extensive research.
Hu: This seems like a valuable combination of traditional research and social data collection!
Zong: That's not all - we then develop AI-powered R&D tools based on these scenarios. Just input your marketing goals - targeting young people, specific sports scenarios, particular ingredients, or flavors - and AI scans through hundreds of scenarios in real-time to identify which consumer needs match, calculating scenario demands to quickly generate vivid consumer need descriptions. These aren't imagined - they come from real scenarios. This step is called needs analysis.
Hu: That's step two - is there a third step?
Zong: After needs analysis, we move to various analyses. For example, for a summer sports scenario with several needs, we select one or combine several to analyze suitable ingredients, searching across the web and our ingredient database. Then we analyze flavors and packaging. Finally, we use AI to generate product concepts, including product concept images - all possible with large language models.
Hu: This is a valuable, complete AI-empowered application from data to final solution!
Zong: We'll soon launch this innovative AI Solution product innovation tool in the food and beverage industry. This represents a completely new logic in insights - combining AI, consumer data, and workflow into a productized solution. It's not just for top clients - innovative enterprises and new consumer brands can use it too. We'll replicate this model across other industries.
09 AI Enables More Actionable Implementation Advice
Hu: You mentioned emotion recognition earlier - what other AI implementation scenarios are you exploring in this area?
Zong: We also help clients generate product background images for e-commerce or other marketing purposes. As a user insight company, we incorporate user emotional needs and product understanding into AI prompts, including many consumer emotional scenario needs, resulting in high-quality output.
Chatbot based on real data sources
Hu: Any specific use cases?
With AI's arrival, the possibilities are endless. However, if we don't focus these possibilities around our strategic direction, it can lead to waste through scattered efforts. By combining various capabilities, we can create highly practical products.
Related articles
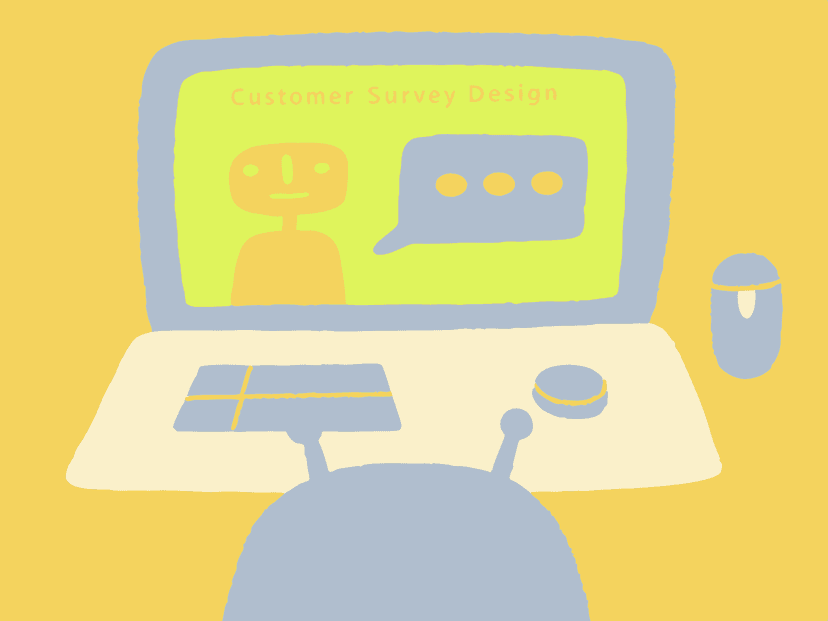
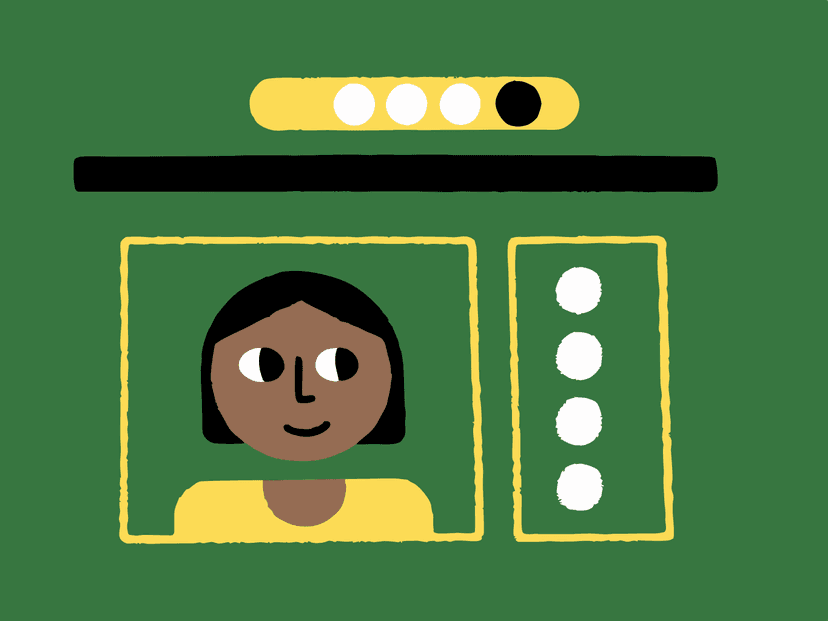
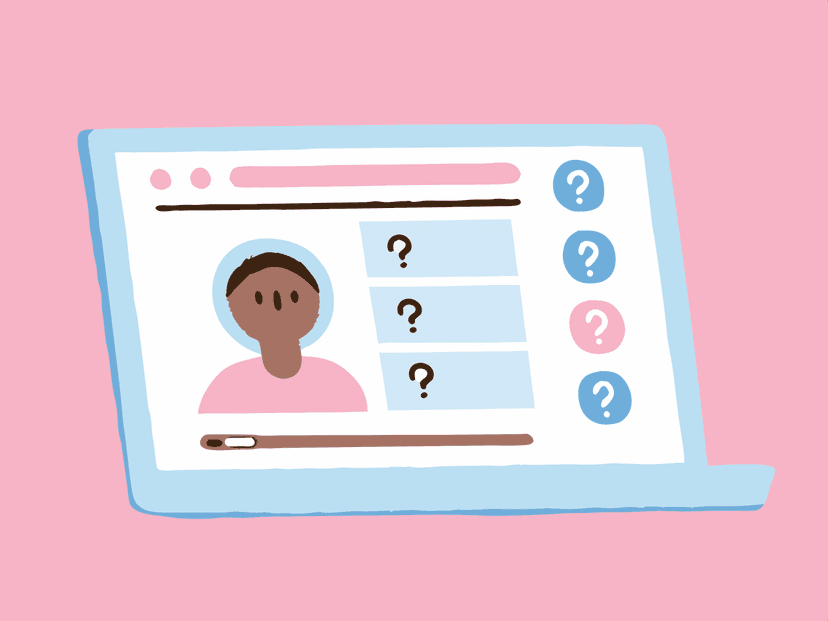
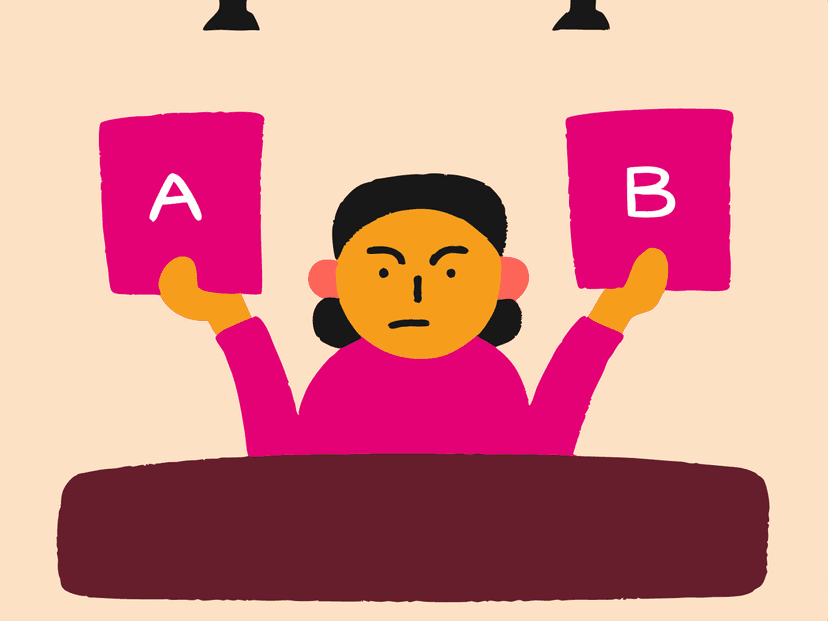