Tuesday, December 3, 2024
Max Diff vs Conjoint Analysis: when to choose the right one
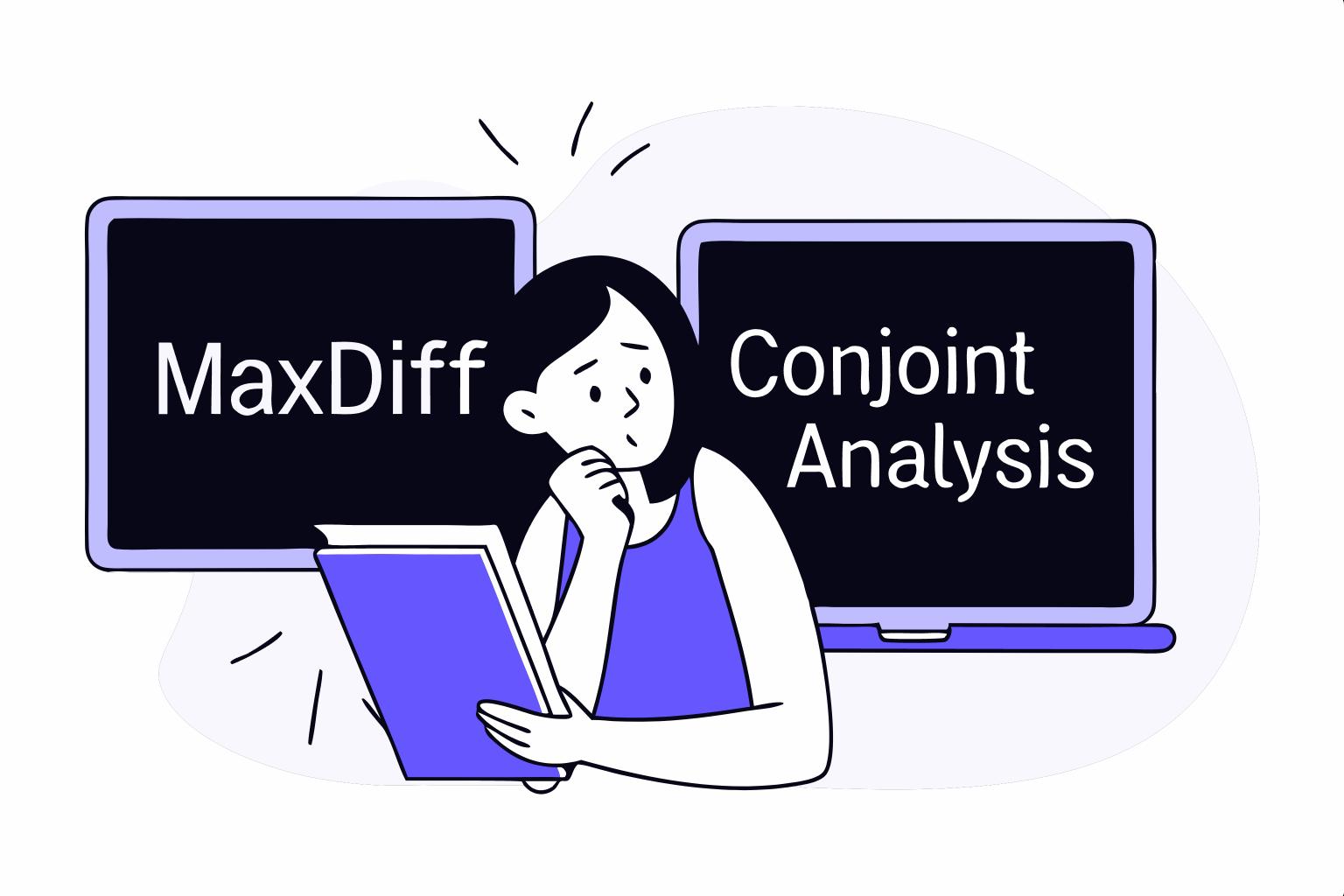
When making critical product and pricing decisions, market researchers often turn to two powerful methodologies: MaxDiff and Conjoint Analysis. While both help uncover customer preferences, they serve distinct purposes and excel in different scenarios. MaxDiff excels at ranking feature importance and preferences, while Conjoint Analysis shines in understanding complex purchase decisions and price sensitivity. This article provides a comprehensive comparison to help you choose the right method for your research needs.
Introduction
MaxDiff and Conjoint Analysis are two powerful research methods used in market research. MaxDiff is a method that asks respondents to choose the "most important" and "least important" items from multiple options, while Conjoint Analysis simulates real purchase scenarios by having users choose between different product combinations.
This article provides a comprehensive comparison of these two methods from aspects including research questions, theoretical differences, implementation costs, and best practices. Reading time is approximately 10 minutes.
Differences in Research Objectives
MaxDiff (Maximum Difference Scaling) is a research method that asks respondents to choose the "most important" and "least important" items from multiple options. This method is particularly suitable for quickly determining user preferences in early product stages.
Conjoint Analysis, on the other hand, is an in-depth research method that simulates real purchase scenarios by having users choose between different product combinations. In practical applications, we can use it to precisely calculate how much users are willing to pay for each feature. This provides scientific basis for our pricing strategy and reasonable support for product premiums.
Imagine ordering at a restaurant. MaxDiff is like a waiter asking: "Among these dishes, which one do you like most and which one do you like least?" Simple and direct, right? Conjoint is more like asking: "Would you choose an 128 Sichuan Boiled Fish with side dishes?" - This requires more thought and trade-offs.
Theoretical Differences
MaxDiff's core is based on Discrete Choice Theory in statistics. The method obtains highly discriminative preference data by forcing respondents to make polarized choices among multiple options. This methodological design cleverly avoids the central tendency bias found in traditional Likert scales.
Imagine you're a food critic with multiple dishes in front of you. MaxDiff is like being asked to clearly indicate your "most liked" and "least liked" choices among these dishes. Behind this seemingly simple choice lies profound statistical principles - Discrete Choice Theory.
This method cleverly avoids the "central tendency" problem common in traditional rating methods (like 1-5 scale ratings). It's like when selecting the best employee - if managers rate everyone, they might give everyone 4 points; but if asked to choose the "most outstanding" and "most needs improvement" employees, the results become much clearer.
Conjoint Analysis originates from mathematical psychology, with its theoretical foundation built on Utility Decomposition. This method breaks down products into independent attributes and levels, then uses orthogonal design to build test combinations, ultimately quantifying the part-worth utilities of each attribute.
Conjoint Analysis works like a smart product designer - its unique strength lies in breaking complex products into basic attributes and testing consumer preferences through different combinations. Based on Utility Decomposition theory, it's like analyzing a delicious meal by evaluating how each ingredient, seasoning, and cooking method contributes to the overall taste. Just as a chef carefully balances flavors, Conjoint Analysis helps businesses understand how different product features work together to create value for customers.
Differences in Implementation Costs
Survey Response Burden and Time Investment
MaxDiff's Simplicity:
-
Simple task: Choose "most important" and "least important"
-
Each decision takes only 10-15 seconds
-
Similar to quick shopping decisions. For example: When testing phone features, respondents completed 8 sets of choices in 2 minutes with minimal fatigue.
Conjoint's Deep Thinking:
-
Requires weighing multiple factors
-
Each decision takes 30-45 seconds
-
Simulates real purchase decisions. For example: When testing phone product combinations, respondents carefully compare price, performance, looks and other features, just like actual shopping.
Survey Design Complexity
MaxDiff Design Features:
-
Relatively simple survey design
-
Focuses on single dimension comparisons
-
Suitable for 5-15 test items. For example: We completed an app feature priority survey design in just half a day.
Conjoint Design Features:
-
Requires careful attribute and level design
-
Considers interactions between factors
-
Usually includes 3-7 attributes with 2-5 levels each. For example: Designing a smartwatch Conjoint study took us a full week to balance price, features, appearance and other dimensions.
Differences in Statistical Analysis Methods and Report Conclusions
For data modeling, MaxDiff uses Multinomial Logistic Regression to estimate parameters. This method produces ratio scale measurements that are significantly better at differentiating than traditional ordinal scale methods.
In actual calculations, we can not only determine the importance ranking of factors but also precisely quantify the differences between them. For example, in a smart home product study, MaxDiff showed that voice control was exactly 2.7 times more important than touch control.
We can also apply TURF (Total Unduplicated Reach and Frequency) analysis for additional insights beyond the MaxDiff results.
Conjoint analysis is based on Random Utility Theory and uses Hierarchical Bayes Estimation to calculate individual-level utility values. This method can estimate attribute importance and predict market share through a Market Simulator. In practice, its prediction accuracy usually reaches a confidence interval of ±5%.
Conjoint analysis handles more complex decision scenarios, mainly using Hierarchical Bayes Regression for parameter estimation. This method can calculate each respondent's personal preference level for different attributes rather than just getting an average across all respondents, making the analysis results more precise and personalized.
Beyond segmented analysis, Conjoint Analysis also supports precise quantification of market share for different attribute combinations. For instance, in a smartphone product study, Conjoint Analysis not only showed that 5G was more popular than 4G but could precisely predict that adding 5G would bring an 18.5% market share increase.
Using individual data, researchers can also identify consumer groups with similar preference patterns through Latent Class Analysis. For example, in the same smartphone study, we found two main segments: "Performance Seekers" (35%) and "Value Seekers" (45%). Performance Seekers were willing to pay 300 more. Such insights directly guide differentiated pricing strategies.
When to Use MaxDiff or Conjoint Analysis
When choosing a research method, consider three key factors: project stage, budget size, and urgency of decisions. Startups with limited budgets should start with MaxDiff. Established companies with mature products are better suited for Conjoint Analysis for detailed decision-making.
Guide to Choosing the Right Analysis Method
Learn more about the differences between MaxDiff and Conjoint Analysis
-
Research Goals
-
If your main goal is understanding how consumers view multiple product/service attributes with 2+ levels each, Conjoint Analysis is your best choice. It provides deep insights into consumer preferences and reveals trade-offs they're willing to make between different attributes.
-
On the other hand, if you need to rank or prioritize a list of items (like features, benefits, or information), MaxDiff analysis is more suitable. It gives clear quantitative data showing what consumers value most and least.
-
-
Product Complexity
-
Conjoint Analysis works especially well for products/services with multiple attributes that consumers must consider simultaneously (like cars, software, or financial services). This method helps determine the best combination of attributes to attract your target audience.
-
MaxDiff analysis suits simpler products or when you need to focus on ranking a list of options, like deciding which features to include in a new product version or identifying the most compelling marketing messages.
-
-
Budget and Time Constraints
- If you have more budget and time for in-depth research, Conjoint Analysis can provide the detailed insights you need. However, if your resources are limited or you need quick results, MaxDiff analysis is a faster, more cost-effective alternative.
Decision Framework
-
Research Goals: Do you want to understand how the levels of multiple attributes lead to product selection (Conjoint) or sorting a list of items (MaxDiff)?
-
Product Complexity: Does your product or service have multiple attributes that need to be evaluated (Conjoint), or is it more direct (MaxDiff)?
-
Budget and Time Constraints: Do you have the time and budget for complex research (Conjoint), or do you need a faster, simpler solution (MaxDiff)?
Conclusion
Choosing the right research method is like choosing the right tool. When we need to make clear choices among existing options, MaxDiff is the best choice; when we need to understand the market performance of product attribute combinations, Conjoint analysis provides a more comprehensive insight. Understanding the characteristics and application scenarios of these two methods can help us make more informed method choices in market research, thereby obtaining more valuable research results.
Finally, remember that no matter how good the method is, it needs to be combined with a reasonable execution plan. It is recommended to clearly define the time nodes and decision goals before the project starts. Choosing the right tool is only half the battle; accurate question design and professional data analysis are also crucial.
Related articles
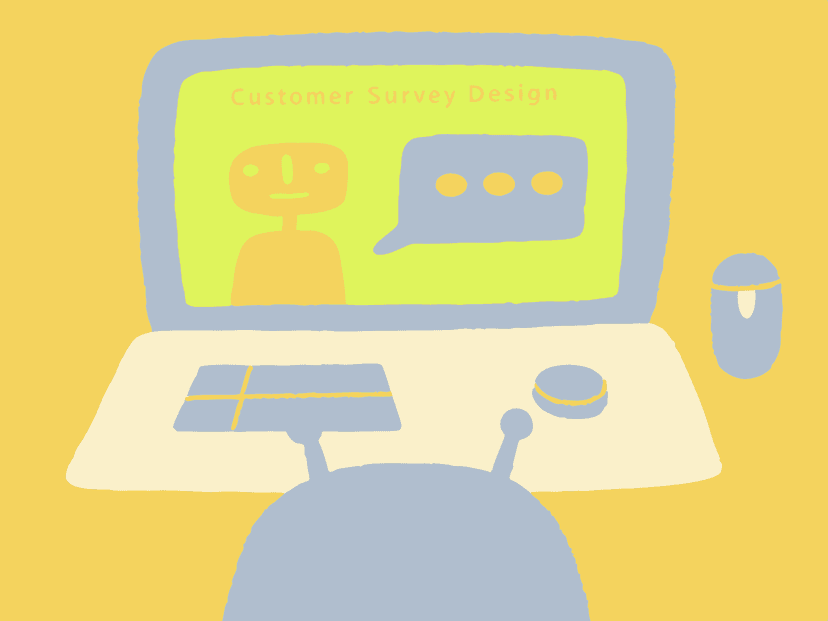
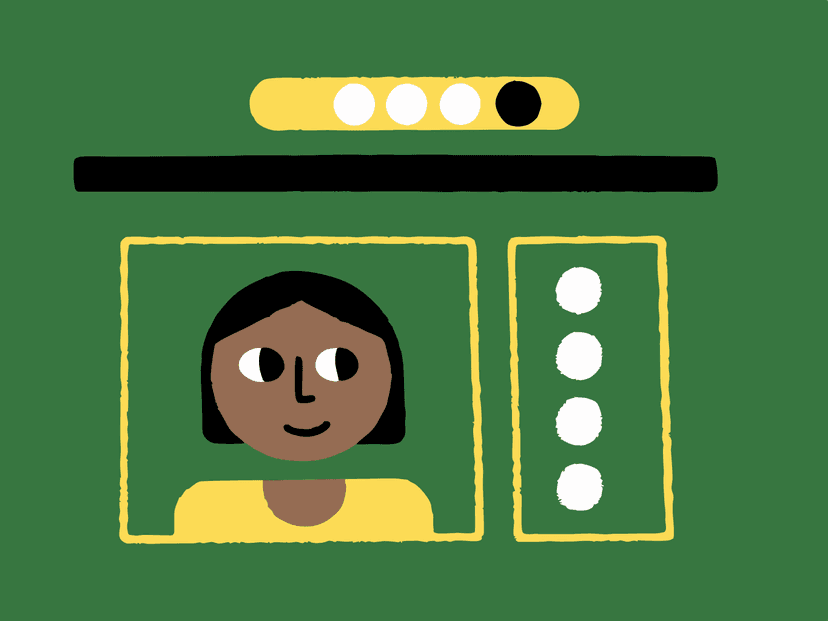
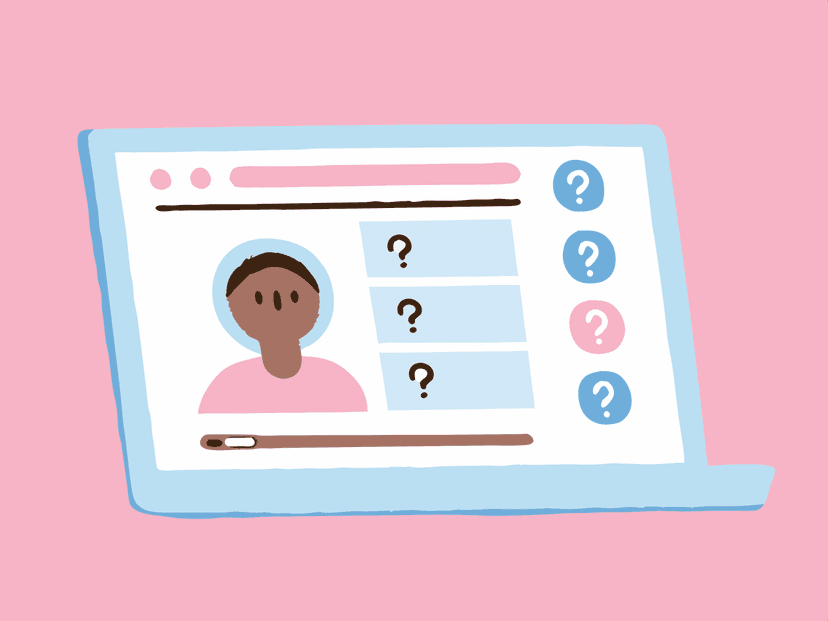
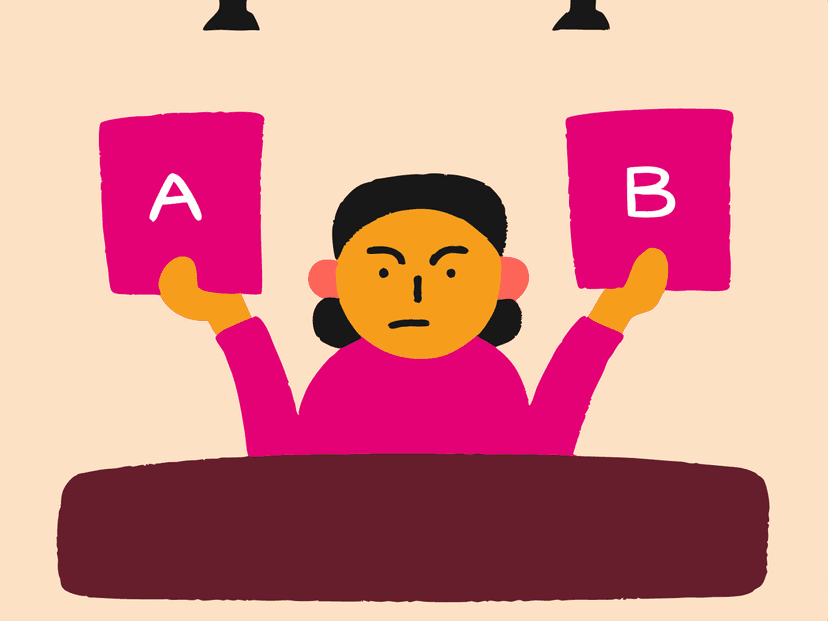