Thursday, December 26, 2024
Quantitative research
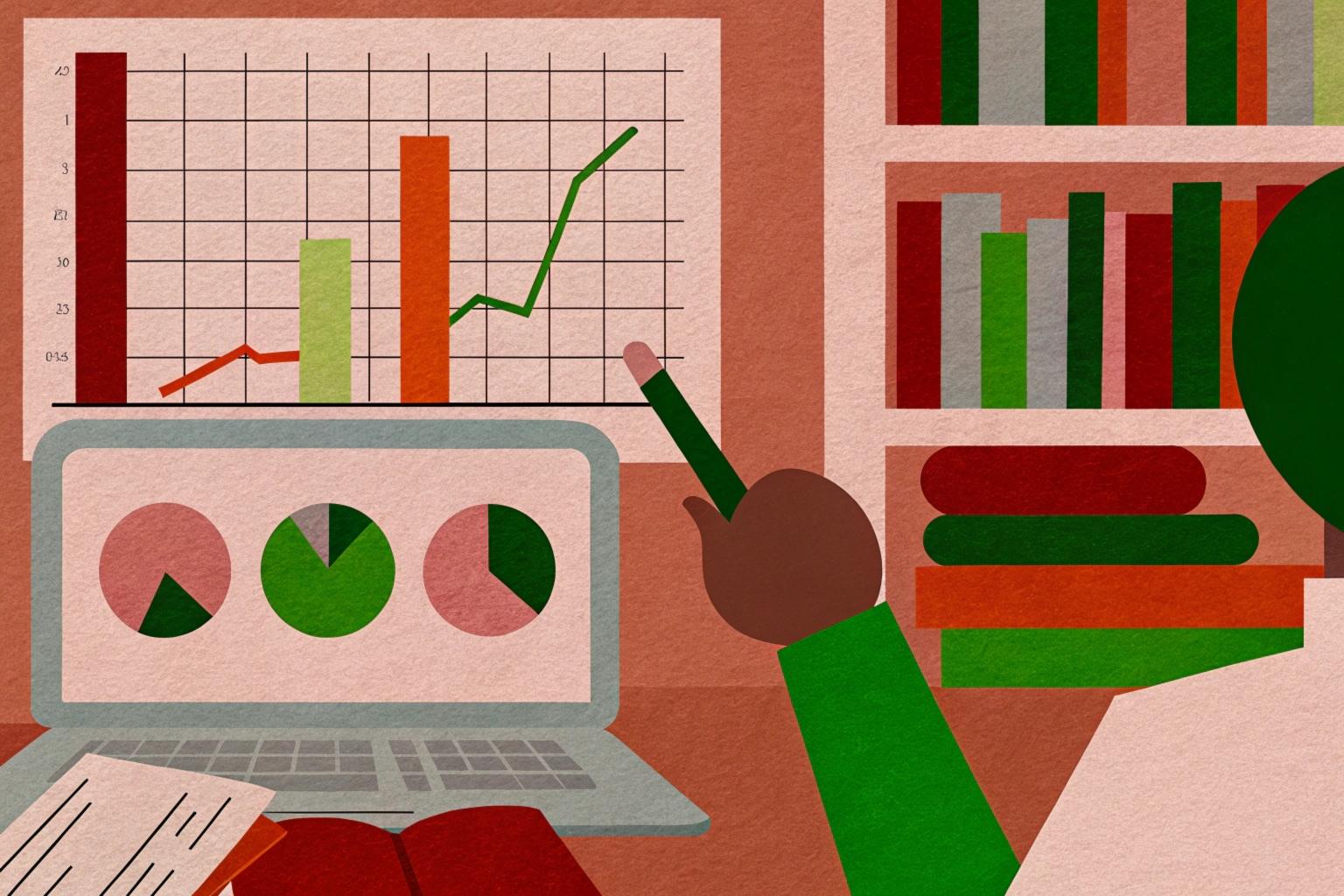
Quantitative research is a systematic and empirical approach to investigating phenomena through numerical data and statistical methods. Unlike qualitative research, which focuses on exploring subjective experiences, quantitative research emphasizes measurement, objectivity, and generalizability. This approach is widely applied in fields such as social sciences, computer science, consumer psychology, economics, and marketing, where quantifiable evidence is essential for hypothesis testing, identifying patterns, and making predictions.
How Different Fields Harness Quantitative Research
Quantitative research plays a pivotal role across various disciplines, each leveraging its strengths to address unique challenges and objectives. In social sciences, it is used to analyze societal trends, human behaviors, and social structures. Bryman (2016) highlights that its reliance on statistical methods, such as surveys, experiments, and longitudinal studies, enables researchers to uncover correlations and causal relationships. For example, quantitative methods help in understanding public opinion, voting behaviors, or demographic shifts across populations.
In computer science, quantitative research is integral to evaluating algorithms, system performance, and computational models. Metrics such as accuracy, precision, recall, and response time are used to measure the effectiveness of machine learning algorithms and other computational systems. This data-driven approach ensures that systems are optimized for reliability and scalability, which is critical in areas like artificial intelligence and cybersecurity.
In consumer psychology, quantitative research is employed to uncover consumer preferences, behaviors, and decision-making processes. Techniques such as structural equation modeling and regression analysis are frequently used to examine relationships between variables like brand loyalty, purchase intent, and satisfaction (Hair et al., 2019). These insights help businesses tailor their marketing strategies and product offerings to meet consumer needs.
In economics, quantitative research is the backbone of econometric modeling and statistical analysis. Economists use quantitative tools to analyze relationships between economic variables, such as inflation, unemployment, and GDP. For instance, Samuelson and Solow's (1960) study on the Phillips Curve used quantitative methods to explore the inverse relationship between inflation and unemployment, providing a foundation for macroeconomic policy development.
In marketing, quantitative research is indispensable for understanding market dynamics, segmenting audiences, and evaluating campaign effectiveness. Techniques such as A/B testing, predictive analytics, and cluster analysis allow marketers to make data-driven decisions. Malhotra et al. (2017) emphasize that quantitative research enables businesses to identify consumer trends, measure brand performance, and optimize marketing strategies to maximize ROI.
How AI is Transforming Quantitative Research
Artificial Intelligence (AI) is reshaping quantitative research by improving data collection, analysis, and interpretation. AI-powered tools provide unprecedented efficiency and scalability, allowing researchers to process vast datasets and uncover insights that were previously inaccessible.
One of the key advancements brought by AI is in data collection. Traditional methods, such as surveys and experiments, often require significant time and resources. AI-driven platforms streamline this process through automated surveys, real-time data gathering, and chatbot interactions. For instance, tools like Qualtrics and SurveyMonkey incorporate AI to improve survey design and ensure higher response rates by customizing questions based on user behavior.
AI also enhances data preprocessing, an essential step in quantitative research. Cleaning large datasets, identifying outliers, and filling missing values can be time-consuming and prone to error. AI algorithms automate these processes, ensuring that datasets are accurate and ready for analysis. This is particularly useful in fields like marketing and consumer psychology, where datasets often include unstructured data from social media or customer reviews.
In data analysis, AI-powered machine learning models are enabling researchers to uncover complex, non-linear relationships between variables. This is particularly evident in predictive modeling, a core aspect of quantitative research. For example, AI algorithms can analyze historical purchasing data to predict future consumer behavior, allowing businesses to optimize inventory and marketing strategies. Similarly, in economics, AI models are used to forecast market trends and assess the potential impact of policy changes.
Additionally, AI is transforming visualization and reporting in quantitative research. Tools like Tableau and Power BI now integrate AI features that generate automated insights and visualizations, making it easier for researchers and decision-makers to interpret complex data. Natural Language Processing (NLP) allows these tools to answer queries in plain language, democratizing access to advanced analytics.
Despite these advancements, the integration of AI into quantitative research raises ethical concerns. Issues such as algorithmic bias, data privacy, and transparency must be addressed to ensure that AI-driven research remains reliable and fair. Moreover, while AI can process and analyze data at an unprecedented scale, human oversight is critical to contextualize findings and avoid misinterpretation.
References
- Bryman, A. (2016). Social Research Methods (5th ed.). Oxford University Press.
- Hair, J. F., Black, W. C., Babin, B. J., & Anderson, R. E. (2019). Multivariate Data Analysis (8th ed.). Cengage Learning.
- Malhotra, N. K., Nunan, D., & Birks, D. F. (2017). Marketing Research: An Applied Approach (5th ed.). Pearson.
- Samuelson, P. A., & Solow, R. M. (1960). Analytical Aspects of Anti-Inflation Policy. The American Economic Review, 50(2), 177–194.